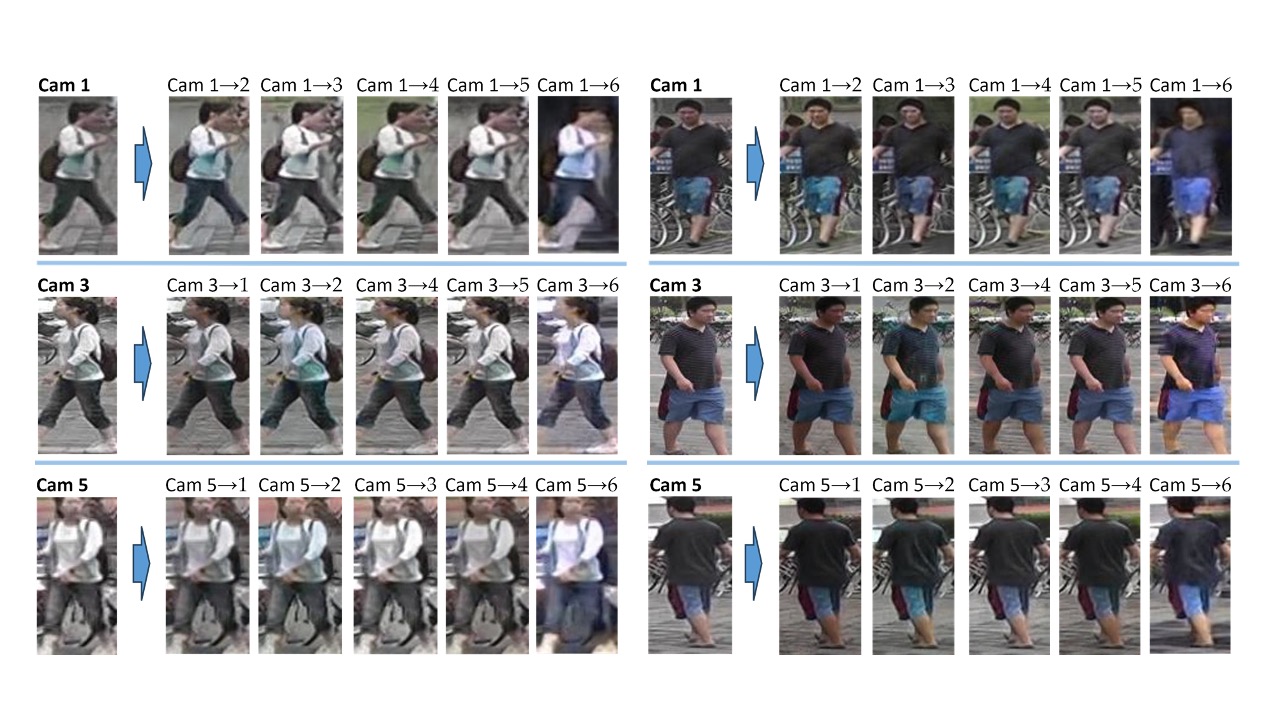
Some style-transferred samples in Market-1501 dataset. Each image, originally taken by a specific camera, is transformed to align with the styles of the other five cameras, both within the training and test data. The real images are shown on the left, while their corresponding style-transferred counterparts are shown on the right.
CORE-ReID ranked 1st on Papers with Code
CORE-ReID, Cybercore’s Unsupervised Domain Adaptation for Person Re-ID algorithm, is ranked in the 1st place, in the relevant category on “Papers with Code”, a site for publishing papers that gather researchers from all over the world.
The algorithm’s name is derived from our company name, CORE in “Cyber Core.” The research was carried out by our AI engineers in collaboration with the PRIMA lab, led by Dr. Prima at Iwate Prefectural University (http://p-www.iwate-pu.ac.jp/~prima/).
Features of CORE-ReID:
The features of CORE-ReID are as follows:
1. Novel Dynamic Fine-Tuning Approach with Camera-Aware Style Transfer using Generative AI: We introduce a pioneering fine-tuning strategy that employs a camera-aware style transfer model for Re-ID data augmentation. This novel approach not only addresses disparities in images captured by different cameras but also mitigates the impact of Convolutional Neural Network (CNN) overfitting on the source domain
2. Innovative Efficient Channel Attention Block (ECAB): We develop a groundbreaking ECAB that leverages the inter-channel relationships of features to guide the model’s attention to meaningful structures within the input image. This innovation enhances feature extraction and focuses the model on critical identity-related features.
3. CORE Framework with Ensemble Fusion of Global and Local Features: We establish the CORE (Comprehensive Optimization and Refinement through Ensemble Fusion) framework, which utilizes a novel pair of teacher-student networks to perform an adaptive fusion of global and local (top and bottom) features for multi-level clustering with the objective of generating diverse pseudo-labels. By proposing the Bidirectional Mean Feature Normalization (BMFN), the model can increase its discriminability at the feature level and address key limitations in existing methods.
Experimental results conducted on three widely used UDA Person Re-ID datasets demonstrate that our CORE-ReID outperforms state-of-the-art approaches in terms of performance.
Paper Title: “CORE-ReID: Comprehensive Optimization and Refinement through Ensemble fusion in Domain Adaptation for person re-identification”.
Related URLs:
Papers with code – Unsupervised Domain Adaptation’s benchmark – https://paperswithcode.com/task/unsupervised-domain-adaptation
Papers with code – https://paperswithcode.com/paper/core-reid-comprehensive-optimization-and
Paper – https://www.mdpi.com/2674-113X/3/2/12
Project Page – https://trinhquocnguyen.github.io/core-reid-homepage